UMD Team Wins Best Paper Award at WACV 2020
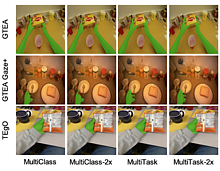
A team of researchers from the University of Maryland recently received a Best Paper award for their work that uses novel applications of computer vision to improve access to information for people who are visually impaired.
“Hand-Priming in Object Localization for Assistive Egocentric Vision,” was recognized at the 2020 Winter Conference on Applications of Computer Vision (WACV 2020), held in March in Aspen, Colorado.
The paper—by Kyungjun Lee, a fourth-year doctoral student in computer science, Abhinav Shrivastava, an assistant professor of computer science with an appointment in the University of Maryland Institute for Advanced Computer Studies, and Hernisa Kacorri, an assistant professor in the College of Information Studies (iSchool)—explores the concept of object localization for egocentric vision.
Egocentric vision, a sub-field of computer vision that entails analyzing images and videos captured by a wearable camera, holds great promises for increasing access to visual information and improving the quality of life for people with visual impairments, the researchers say.
Object recognition—is that a jar of nutmeg or red pepper?—is one of the daily challenges for this population.
While researchers strive to improve recognition performance, it remains difficult to identify which object is of interest to the user; the object may not even be included in the frame due to challenges in camera aiming without visual feedback. Also, gaze information, commonly used to infer the area of interest in egocentric vision, is often not dependable. However, blind users often tend to include their hand either interacting with the object that they wish to recognize or simply placing it in proximity for better camera aiming.
In the paper, the team proposes localization models that leverage the presence of the hand as the contextual information for priming the center area of the object of interest.
Their evaluation demonstrates the effectiveness of using the hand segmentation feedback for object localization—estimating the center area of a target object. It also shows that explicit infusion of the hand information into an object localization network achieves more precise localization than other approaches.
The researchers believe their method can be further employed in other applications that need to understand hand–object interactions, such as object/action recognition and assistive systems for people with visual impairments.
—Story by Melissa Brachfeld