UMD Study Validates Face Recognition Experts, But Shows Humans Perform Best with an AI Partner
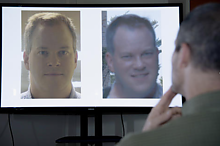
New research that combines computer vision research, forensic science, and psychology shows that experts in facial identification are highly accurate, but that the highest accuracy in face recognition comes through the partnering of a human expert with state-of-the-art face recognition software.
A team of scientists from the University of Maryland, the National Institute of Standards and Technology, the University of Texas at Dallas, and the University of New South Wales tested and compared the face recognition accuracy of forensic examiners, computer face recognition programs, and people with no training in face recognition. A paper based on the research was published May 29, 2018, in the journal Proceedings of the National Academy of Sciences (PNAS).
The study, part of efforts to strengthen forensic science in the U.S., found that the performance of professionally trained facial identification experts was much more accurate than that of people untrained in facial recognition. And it showed this accuracy was further enhanced by combining the evaluations of multiple experts, a common forensic practice.
However, UMD Distinguished University Professor Rama Chellappa, a study co-author and nationally recognized leader in computer face recognition, said that the other two main results were more surprising.
“We found that the face recognition performance of the best computer algorithms is up there with the performance of forensic examiners,” said Chellappa, who is a Minta Martin Professor of Engineering and chair of the Department of Electrical and Computer Engineering and a leading computer vision researcher in the University of Maryland Institute for Advanced Computer Studies (UMIACS).
Study co-author and UMIACS Assistant Research Scientist Carlos Castillo said: “This finding represents a computer achievement comparable to the chess playing performance of IBM’s Deep Blue in matches [1996–1997] with then-World Chess Champion Garry Kasparov.”
“We don’t know yet how this finding should be implemented in forensic practices, but it appears that computer face recognition is a tool that forensic science can use,” said Chellappa, whose postdoctoral associate Jun-Cheng Chen and two doctoral students, Rajeev Ranjan and Swami Sankaranarayanan, designed and developed the three face recognition programs used in the study and were co-authors for the study.
The top performing program, A2017b, whose inventors are Ranjan, Castillo and Chellappa, was named a UMD Invention of the Year in April.
According to Chellappa, the broader context for the study is that it is a step in the process of learning how machine and humans can best work together. “These findings add to such knowledge and to the possibility that humans can trust machines to help them.”
The team’s effort began in response to a 2009 report by the National Research Council, Strengthening Forensic Science in the United States: A Path Forward, which underscored the need to measure the accuracy of forensic examiner decisions. In their recent study published in PNAS, the researchers note that remarkably little was previously known about the accuracy of forensic facial comparisons by examiners relative to such comparisons by people without training, and nothing was known about their accuracy relative to computer-based face recognition systems.
“This is the first study to measure face identification accuracy for professional forensic facial examiners, working under circumstances that apply in real-world casework,” said NIST electronic engineer and first author P. Jonathon Phillips. “Our deeper goal was to find better ways to increase the accuracy of forensic facial comparisons.”
The study involved a total of 184 participants. Fifty-seven were forensic facial examiners, the experts who get the highest level of professional training in the identification of faces in images and videos. Forensic facial examiners provide identification evidence in police investigations and in criminal trials. Thirty study participants were facial reviewers, who get a lower level of training in facial identification. Thirteen were “super recognizers,” people with exceptional natural ability to recognize faces. The remaining 84—the control groups—included 53 fingerprint examiners and 31 undergraduate students, none of whom had training in facial comparisons.
For the test, the participants received 20 pairs of face images and rated the likelihood of each pair being the same person on a seven-point scale. The research team intentionally selected extremely challenging pairs, using images taken with limited control of illumination, expression and appearance. They then tested four of the latest computerized facial recognition algorithms, all developed between 2015 and 2017, using the same image pairs.