UMD Team Is Finalist for Prestigious ACM Gordon Bell Prize
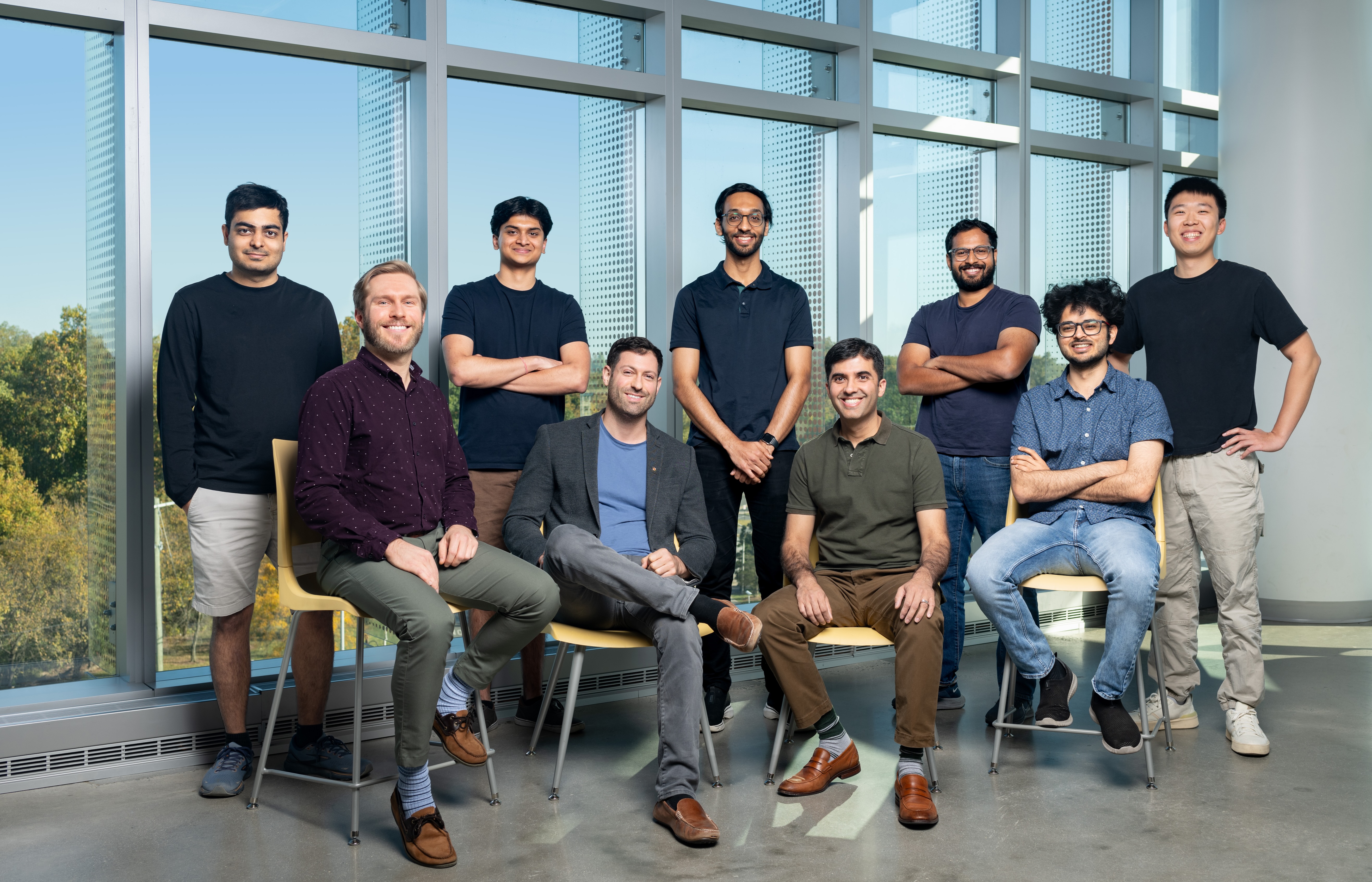
A team of parallel computing and machine learning experts from the University of Maryland has been named a finalist in a global competition recognizing outstanding contributions in high-performance computing.
The UMD team—led by Abhinav Bhatele, an associate professor of computer science, and Tom Goldstein, a professor of computer science—is one of six vying for top honors in this year’s Association for Computing Machinery’s (ACM) Gordon Bell Prize competition.
The prize honors the late Gordon Bell, a noted expert in high-performance and parallel computing who died in 2024. It comes with a $10,000 award from Bell’s estate and celebrates research and scholarship in high-performance computing that can be applied to science, engineering and large-scale data analytics.
The UMD researchers made the final cut for their innovative work in developing a scalable distributed training framework called AxoNN, which rapidly trains AI-based large language models, or LLMs, on thousands of GPUs.
LLMs are the computational models that drive chat bots such as Open AI’s ChatGPT, Microsoft’s CoPilot and other popular platforms, and are based on billions, if not trillions, of numbers called parameters, or weights—values of which help determine how important a word or piece of information is, and how best to answer questions.
The UMD team’s exascale framework enables AI model training that that scales efficiently and portably on several GPU-based supercomputers, while also addressing key privacy concerns, says Bhatele.
With support from the U.S. Department of Energy, the UMD team tested their algorithm on some of the world’s fastest supercomputers, including the exascale-class Frontier supercomputer at the Department of Energy’s Oak Ridge National Laboratory in Tennessee.
This high-performance platform allowed the UMD team to test their framework’s scalability and efficiency, enabling AxoNN to successfully benchmark AI model training with up to 320 billion parameters at record-breaking exaflop speeds, says Bhatele.
“Training or fine-tuning LLMs requires tremendous amounts of computing resources, and leveraging powerful GPU accelerators in exascale machines is the key to developing the models faster and more efficiently,” he says.
Frontier’s computing power also allowed the Maryland team to study LLM memorization behavior at a larger scale than ever before, says Goldstein, who is the director of the University of Maryland Center for Machine Learning.
The UMD researchers found that privacy risks associated with problematic memorization tend to occur in models with greater than 70 billion parameters.
“Generally, the more training parameters there are, the better the LLM will be,” Goldstein explained. “However, introducing more training parameters also increases privacy issues and copyright risks caused by memorization of training data that we don’t want the LLMs to regurgitate.”
He says the UMD team coined a term for it: “catastrophic memorization.”
To mitigate the problem, Goldstein says, the researchers used a technique called Goldfish Loss that randomly omits certain information during training and prevents the model from memorizing entire sequences that could contain sensitive or proprietary information.
In addition to Bhatele and Goldstein, other team members include UMD computer science graduate students Siddharth Singh, Prajwal Singhania, Aditya Ranjan, John Kirchenbauer, Yuxin Wen, Neel Jain, Abhimanyu Hans and Manli Shu.
Jonas Geiping, a former UMD postdoc who is now working as a research scientist at the Max Planck Institute for Intelligent Systems in Germany, and Aditya Tomar, an undergraduate at the University of California, Berkeley, are also contributing to the project.
Bhatele credits Singh, a fifth-year doctoral student he advises, for laying the groundwork of the project by developing a deep-learning framework that reduces GPU idling by four times and maximizes hardware efficiency.
Singh will present the team’s work at the upcoming International Conference for High Performance Computing, Networking, Storage and Analysis (SC24), where the Gordon Bell prize winner will also be announced.
The Gordon Bell prize finalists often draw the largest crowds, says Singh, who is eager to present his team’s work to an audience of the world’s top researchers in high-performance computing.
“The rooms are jam packed with people. Sometimes, people are standing because they don't even get seats,” he says. “It’s very exciting and it’s an honor for me, personally, as well.”
— Story by UMIACS communications group, with additional reporting by science communicators at Oak Ridge National Laboratory