Resnik Receives NSF Funding to Analyze Text Responses in COVID-19 Survey Data
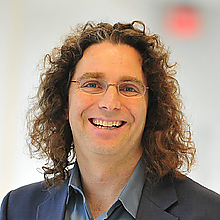
A University of Maryland expert in computational linguistics has received funding from the National Science Foundation (NSF) to develop advanced topic modeling methods that can quickly and effectively analyze text responses in COVID-19 survey data.
Philip Resnik, a professor of linguistics with a joint appointment in the University of Maryland Institute for Advanced Computer Studies, is principal investigator of the $177K award, which comes from the NSF Rapid Response Research initiative.
Resnik plans to collaborate on the project with researchers at the Centers for Disease Control and Prevention’s National Center for Health Statistics, faculty at New York University who are conducting surveys of front-line healthcare providers, and a national coalition of mental health crisis response organizations.
“Good decisions depend on good information, and right now many organizations are using surveys to inform crucial responses and policy choices,” Resnik says. “We’re advancing and deploying computational methods that are aimed at enriching and improving the quality of survey data by taking better advantage of open-ended survey questions—not just traditional multiple choice, but also questions that allow natural, freely generated text responses.”
The project includes two graduate students in the Computational Linguistics and Information Processing Lab, Alexander Hoyle, a first-year doctoral student in computer science, and Pranav Goel, a second-year doctoral student in computer science.
Resnik says a key problem his team will address is analyzing unstructured language in open-ended responses, which is currently a labor-intensive process, creating obstacles to using them especially when speedy analysis is needed and resources are limited. Computational methods can help, Resnik says, but they often fail to provide coherent, interpretable categories, or they can fail to do a good job connecting the text in the survey with the closed-end responses.
The research team’s new technical approach will build on recent techniques that bring together deep learning and Bayesian topic models. Several key technical innovations are being introduced that are specifically geared toward improving the quality of information available in surveys that include both closed- and open-ended responses.
Project activities will include assisting in the analysis of organizations’ survey data, conducting independent surveys aligned with their needs to obtain additional relevant data, and the public release of a clean, easy to use computational toolkit facilitating more widespread adoption of these new methods.
—Story by Melissa Brachfeld