Hartree Fellow Identifies Strengths and Limits of Quantum Computers
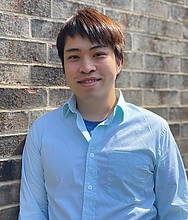
“Quantum” has become go-to technobabble in science fiction movies and TV shows. It’s used to explain away any number of magical plot devices: a quantum tunnel is a time-machine, a quantum fissure is a hole to another reality—you get the idea.
While fiction frequently takes the power of quantum phenomena to fantastical levels, quantum computers do actually have the potential to harness arcane powers to deliver results that would be impossible for even the most powerful “regular” supercomputers. Nai-Hui Chia, a Hartree Postdoctoral Fellow at the Joint Center for Quantum Information and Computer Science (QuICS), explores this territory and identifies the boundaries between where quantum computers reign supreme and where traditional computers can hold their own.
“Because we have quantum computers, computer science becomes totally different,” Chia says. “Quantum computers can do some things better than classical computers, but they cannot do everything better than classical computers. So, I want to know what the landscape of quantum computer science will be like.”
Currently, quantum computers are still limited by their size and by being prone to errors, but researchers are already taking the first steps to demonstrate where these limited quantum computers can deliver advantages. Chia’s research helps identify which computational problems benefit from quantum approaches and which might best be solved with classical computers.
Chia says that he is excited to be researching quantum computer science at this pivotal point when quantum computing is becoming a practical reality, but his journey to studying quantum computation began with him taking an uncertain path as an undergraduate at National Taiwan University (NTU).
“I was a fan of Richard Feynman since I was a student in junior high school,” Chia says. “So, my first dream was to become a physicist. But when I went to university, I didn't get into the physics department; I got into the computer science department.”
Being in that department didn’t derail his interest in physics. He eventually stumbled on an opportunity to combine computer science and physics.
“One day my friend told me about quantum computers, so I read some papers and found that okay, this is interesting,” Chia says. “But at that time in Taiwan, very few people knew quantum computers. When I decided to do research in quantum computers, lots of people told me that I shouldn't do that.”
He was able to take a course about quantum computing in his third year at NTU in the physics department. The following year he joined the lab of Sy-Yen Guo in the electrical engineering department, where he studied the subject by reading textbooks and discussing it with graduate students.
“It was not easy to maintain my interest in quantum computing,” Chia says. “I did not know who was working in this area and which universities to apply to. I was almost about to leave the area and do my Ph.D. in machine learning or data mining.”
Chia applied to graduate school for computer science at several universities in the U.S., ultimately ending up at Pennsylvania State University in 2012. As he contemplated focusing on other areas of computer science, he took an advanced algorithms class with the computer scientist Sean Hallgren and arranged to explore quantum algorithms with him for his graduate research.
They hoped to develop an algorithm to be a powerful tool in the field of cryptography—the field of making and breaking codes that forms the backbone of internet and computer security. While their first attempt to design a powerful quantum algorithm hit many roadblocks, the repeated failed approaches turned into a proof that the attempts were fruitless: The algorithm they were searching for wasn’t possible. The experience opened Chia’s eyes to the importance of identifying and rigorously defining what quantum computers can and can’t do.
He went on to do postdoctoral research at The University of Texas at Austin with Scott Aaronson, who researches the capabilities and limits of quantum computers. While working with Aaronson, he collaborated with early-career colleagues in Taiwan who had had returned after studying abroad. Together they investigated the capabilities of small quantum computers working in tandem with traditional computers and compared their problem-solving prowess to the large, general-purpose quantum computers that researchers dream of.
In particular, they investigated how the size of small quantum computers effects the advantage that they can deliver. They studied the size in terms of the quantum depth—the greatest number of discrete steps, or computational gates, that can occur in the connected collection of gates that make a quantum circuit. The quantum depth of a circuit is not just limited by how many individual quantum bits—called qubits—there are. A qubit might be used multiple times in a circuit, but the depth can be limited by the effect of errors building up with each use of a qubit. Theories suggest that certain problems require a specific level of quantum depth for quantum machines to offer an advantage.
In 2005, Richard Jozsa, then a computer scientist at the University of Bristol, conjectured that a quantum algorithm that called for a large quantum depth could be run using a system with a smaller quantum depth by offloading parts of the computation to a classical computer. However, this idea was never formally proven and others, including Aaronson, suspected there was a separation relative to an oracle.
Chia and his colleagues proved that for specific types of problems—the whimsically named oracle problems—this hybrid approach cannot deliver the advantages promised by larger quantum computers. Their proof established that a general quantum computer has advantages over a hybrid computer for certain tasks.
“We can show that more quantum depth gives you more powerful quantum computers,” Chia says. “Our work tells people that we probably still need general quantum computers to have full quantum power. But this is only a theoretical problem. So, this is just a first step to proving it. We will also need to have a practical problem that can show that the power of a general quantum computer is much better than a hybrid one.”
While at UT, he also investigated the possibility that some of the predicted advantages of quantum computers might be a mirage. Specifically, he looked at algorithms developed in the field of machine learning—a type of artificial intelligence where a computer algorithm improves itself at a task based on exposure to prepared data. Researchers have been trying to develop quantum machine learning algorithms to take advantage of a quantum computer’s ability to use the phenomena of quantum superposition and entanglement to solve certain problems exponentially faster.
But Chia and colleagues showed that for many problems the quantum machine learning algorithms that researchers have developed fail to deliver practical benefits when compared to the right non-quantum approach. They showed that some limitations of quantum computing, like the increased difficulty of getting data into and out of a quantum computer compared to a classical computer, proved to be obstacles to dominating the computational race.
But their result still highlights the value of the quantum algorithms researchers had already proposed. The researchers developed a way of “dequantitizing” the algorithms to create quantum-inspired algorithms that provide new ways to efficiently solve the problems using traditional computers.
This procedure involves designing the algorithm so that the data is given to a classical computer in a structured way that mimics how a quantum computer would access it. With that structure and the greater flexibility of classical computers for entering and retrieving data, Chia and colleagues were able to demonstrate a framework for taking a quantum algorithm for specific types of problems and making a non-quantum version that works at a similar speed.
Chia says that he is optimistic that researchers will still find problems that will benefit from quantum machine learning approaches even though their work has revealed that some problems just required a different classical approach.
“To show the power of quantum computers we need to find new problems, and we learned something from this process,” Chia says. “We can probably find new problems that can really achieve quantum exponential speed up in machine learning.”
—Story by Bailey Bedford
QuICS is a partnership between the University of Maryland and the National Institute of Standards and Technology. The center receives technical and administrative support from the University of Maryland Institute for Advanced Computer Studies.